Translate
lunes, 31 de diciembre de 2018
Minilibros gratuitos.
Quiero compartir estos 2 libros gratuitos de Java Code Geeks :
Download Minibooks!
jueves, 27 de diciembre de 2018
The Hundred-Page Machine Learning Book
Podemos bajarnos el libro "The Hundred-Page Machine Learning Book" la versión draf hasta que sea publicado.
Sin más dejo el link: http://themlbook.com/wiki/doku.php
lunes, 24 de diciembre de 2018
Feliz Navidad!!
Desde el blog queremos desearle una muy Feliz Navidad!!!
nano navidad.sh
#!/bin/bash
trap "tput reset; tput cnorm; exit" 2
clear
tput civis
lin=2
col=$(($(tput cols) / 2))
c=$((col-1))
est=$((c-2))
color=0
tput setaf 2; tput bold
# Tree
for ((i=1; i<20; i+=2))
{
tput cup $lin $col
for ((j=1; j<=i; j++))
{
echo -n \*
}
let lin++
let col--
}
tput sgr0; tput setaf 3
# Trunk
for ((i=1; i<=2; i++))
{
tput cup $((lin++)) $c
echo 'mWm'
}
new_year=$(date +'%Y')
let new_year++
tput setaf 1; tput bold
tput cup $lin $((c - 6)); echo FELICES FIESTAS
tput cup $((lin + 1)) $((c - 9)); echo Y mucho CODIGO en $new_year
let c++
k=1
# Lights and decorations
while true; do
for ((i=1; i<=35; i++)) {
# Turn off the lights
[ $k -gt 1 ] && {
tput setaf 2; tput bold
tput cup ${line[$[k-1]$i]} {column[$[k-1]$i]}; echo \*
unset line[$[k-1]$i]; unset column[$[k-1]$i] # Array cleanup
}
li=$((RANDOM % 9 + 3))
start=$((c-li+2))
co=$((RANDOM % (li-2) * 2 + 1 + start))
tput setaf $color; tput bold # Switch colors
tput cup $li $co
echo o
line[$k$i]=$li
column[$k$i]=$co
color=$(((color+1)%8))
# Flashing text
sh=1
for l in C O D I G O
do
tput cup $((lin+1)) $((c-3+sh))
echo $l
let sh++
sleep 0.01
done
}
k=$((k % 2 + 1))
done
Y ahora ejecutamos:
bash navidad.sh
domingo, 23 de diciembre de 2018
Operaciones comunes con collections en Kotlin
Vamos a revisar el conjunto de funciones que tiene kotlin para manejo de colecciones:
Vamos a empezar con 'filter'. Éste filtra el contenido de la lista y mantiene solo los elementos que satisfacen el predicado. Por ejemplo, aquí tenemos el predicado que comprueba que un número es par, y solo los números pares están presentes en la lista resultante.
val originalList = listOf(1, 2, 3, 4, 5, 6)
assertEquals(listOf(2, 4, 6), originalList.filter { it % 2 == 0 })
La función map() transforma una colección en una nueva, y transforma cada elemento en esta colección. Por ejemplo, aquí encontramos un cuadrado de cada número dado y la colección resultante es una colección de cuadrados. Tenga en cuenta que la colección resultante contiene tantos elementos como el primero.
val nums = listOf(1, 2, 3, 4, 5, 6)
val nums2 = nums.map { e -> e * 2 }
println(nums2)
Hay varios predicados que verifican si los hechos dados son verdaderos para los elementos. Por ejemplo, 'any' comprueba que hay al menos un elemento que satisface el predicado dado. all verifica si todos los elementos satisfacen el predicado, y none comprueba que ninguno de los elementos satisface el predicado dado.
val nums = listOf(4, 5, 3, 2, -1, 7, 6, 8, 9)
val r = nums.any { e -> e > 10 }
if (r) println("There is a value greater than ten")
else println("There is no value greater than ten")
r = nums.all { e -> e > 0 }
if (r) println("nums list contains only positive values")
else println("nums list does not contain only positive values")
r = nums.none { e -> e > 0 }
if (!r) println("nums list contains only positive values")
else println("nums list does not contain only positive values")
find() encuentra un elemento que satisface el predicado dado y lo devuelve como resultado. Si no hay ningún elemento (obligatorio) que devuelve null.
firstOrNull() busca el primer elemento te devuelve un elemento o 'null' como resultado. Mientras que first() solo toma un predicado y lanza una excepción si no hay tal elemento
val originalList = listOf(1, 2, 3, 4, 5, 6, 7, 8, 9, 10)
val result = originalList.firstOrNull { it > 4 }
assertEquals(result, 5)
count() cuenta el número de elementos que satisfacen el predicado dado. max retorna el máximo, min el mínimo:
val nums = listOf(11, 5, 3, 8, 1, 9, 6, 2)
val len = nums.count()
val max = nums.max()
val min = nums.min()
val msg = """
max: $max, min: $min,
count: $len
"""
println(msg.trimIndent())
partition divide la colección en dos (colecciones) los que cumplen o no un predicado.
val nums = listOf(4, -5, 3, 2, -1, 7, -6, 8, 9)
val (nums2, nums3) = nums.partition { e -> e < 0 }
println(nums2)
println(nums3)
Si desea dividir la colección en varios grupos por clave dada, puede usar groupBy utilizando como argumento la forma de agrupar los elementos, cuál debería ser la clave. Por ejemplo
val nums = listOf(1, 2, 3, 4, 5, 6, 7, 8)
val res = nums.groupBy { if (it % 2 == 0) "even" else "odd" }
println(res)
AssociateBy también realiza la agrupación, pero devuelve un elemento como el valor del mapa. AssociateBy debe usarse solo si la clave es única. Si no es así, se eliminan los duplicados. De esta forma nos quedamos con el ultimo.
val nums = listOf(1, 2, 3, 4, 5, 6, 7, 8)
nums.associate { (if (it % 2 == 0) "greatest_even" else "greatest_odd") to it }
Otra forma de organizar de alguna manera un par de colecciones es zip. El cual las une :
val names = listOf("Jon", "John", "Jane")
val ages = listOf(23, 32, 28)
names.zip(ages)
// [(Jon, 23), (John, 32), (Jane, 28)]
val customerMap = mapOf(Pair(Customer("Jack", 25), Address("NANTERRE CT", "77471")),
Pair(Customer("Mary", 37), Address("W NORMA ST", "77009")),
Pair(Customer("Peter", 18), Address("S NUGENT AVE", "77571")),
Pair(Customer("Amos", 23), Address("E NAVAHO TRL", "77449")),
Pair(Customer("Craig", 45), Address("AVE N", "77587")))
val customerList = customerMap.flatMap { (customer, _) -> listOf(customer) }
customerList.forEach{ println(it) }
/*
Customer(name=Jack, age=25)
Customer(name=Mary, age=37)
Customer(name=Peter, age=18)
Customer(name=Amos, age=23)
Customer(name=Craig, age=45)
*/
Y a veces tenemos una lista de solo elementos como resultado. Entonces, lo que hace la función flatten, toma una lista de listas de elementos y la aplana retorna una lista plana
val list = listOf(
listOf(1, 2, 3),
listOf("one", "two", "three"),
listOf(Customer("Jack", 25), Customer("Peter", 31), Customer("Mary", 18))
)
var flattenList = list.flatten()
println(flattenList)
/*
[1, 2, 3, one, two, three, Customer(name=Jack, age=25), Customer(name=Peter, age=31), Customer(name=Mary, age=18)]
*/
Dejo link: https://kotlinlang.org/api/latest/jvm/stdlib/kotlin.collections/
jueves, 20 de diciembre de 2018
70 libros gratuitos de javascript.
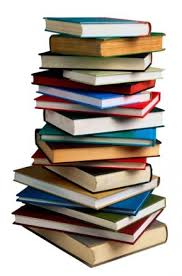
Ya que estoy recomendado libros quiero recomendarle este sitio donde pueden bajar varios libros sobre tecnologías que se utilizan en el front-end.
Dejo link: http://on.edupioneer.net/38ae0c8df8
Libro gratuito de CouchDb, HTML 5, JAXB y JavaFx
Quiero recomendar estos Libros gratuitos:
Download Dev Guides!
|
| ||||||
|
|
miércoles, 19 de diciembre de 2018
Herramientas Open Source front-end para Apache HBase.
Las herramientas open source que se pueden utilizar como clientes de Apache HBase son :
Apache Impala: Antes
una herramienta de Cloudera, pero la han liberado en el marco de la
organización apache. Impala es una herramienta de procesamiento de
consultas en paralelo, utiliza map-reduce y se pueden hacer consultas
a hbase o a archivos hdfs (como hive)
- Visualización de datos.
- Creación, eliminación y modificación de tablas y filas.
- Tablas estaticas
- Scans
Toad for Cloud
Databases: Es una herramienta muy versátil para diferentes productos
entre los que se encuentra hbase.
HareDB HBase Client:
Es un cliente de Hbase que tiene una interfaz amigable.
Hrider: Es una
aplicación super amigable que permite consultar y manipular datos de
hbase.
Hannibal: Es una
herramienta para monitoreo de regiones.
Performance
Monitoring & Alerting (SPM): SPM es una herramienta de monitoreo,
muy completa, tiene la filosofia de detección temprana de los
problemas. A la vez permite monitorear una amplia gama de productos
en los que podemos nombrar : Solr, Elasticsearch, Hadoop, HBase,
ZooKeeper, Kafka, Storm, Redis, JVM, etc.
Apache Phoenix: Es un
cliente embebido que permite consultas como si fuera un driver JDBC. Apache Phoenix permite OLTP y el análisis operativo en Hadoop para aplicaciones de baja latencia combinando lo mejor de ambos mundos: el poder de las API de SQL y JDBC estándar con capacidades de transacción ACID completa y
la flexibilidad de las capacidades de lectura y escritura de los últimos tiempos del mundo NoSQL aprovechando HBase como su tienda de respaldo; Apache Phoenix está totalmente integrado con otros productos de Hadoop como Spark, Hive, Pig, Flume y Map Reduce.
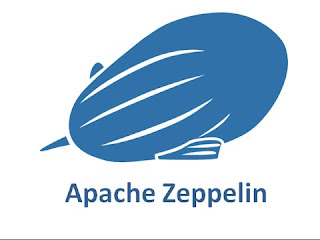
Apache Zeppelin: Notebook basado en la web que permite a los datos,
Análisis de datos interactivos y documentos de colaboración con SQL, Scala y más.
viernes, 14 de diciembre de 2018
Introducing the AI Transformation Playbook
Me llego este mail de Andrew Ng que es el profesor de un curso en coursera sobre machine learning y quiero compartirlo con ustedes ya que me resulto por demás interesante :
| ||||||||||
| ||||||||||
|
jueves, 13 de diciembre de 2018
Libro gratuitos sobre Linux, postgresql, jetty, jdbc
Download Dev Guides!
|
| ||||||
|
|
martes, 11 de diciembre de 2018
¿Como esta compuesto Apache HBase? Parte 4
En la ultima parte hablaremos de 2 componentes: Client y Catalog Table :
El Client es responsable de encontrar el RegionServer, que alberga la fila particular (datos). Se realiza consultando las tablas del catálogo. Una vez que se encuentra la región, el cliente se pone en contacto directamente con RegionServers y realiza la operación de datos. Una vez que se obtiene esta información, el cliente la almacena en caché para una recuperación más rápida. El cliente puede escribirse en Java o en cualquier otro lenguaje mediante API externas.
Las catalog tables son dos tablas que mantienen la información sobre todos los RegionServers y las regiones. Estas son un tipo de metadatos para el clúster HBase. Las siguientes son las dos tablas de catálogo que existen en HBase:
- -ROOT-: Esto incluye información sobre la ubicación de la tabla .META.
- .META. : Esta tabla contiene todas las regiones y sus ubicaciones.
Al comienzo del proceso de inicio, la ubicación .META se establece en la raíz desde donde se leen los metadatos reales de las tablas y la lectura / escritura continúa. Por lo tanto, cada vez que un cliente desea conectarse a HBase y leer o escribir en la tabla, estas dos tablas se remiten y la información se devuelve al cliente para lectura directa y escritura en los RegionServers y las regiones de la tabla específica.
¿Como esta compuesto Apache HBase? Parte 3
Ahora hablaremos del RegionServer que con ese nombre suena importante.
De la misma manera que en clúster de Hadoop, un NameNode administra los metadatos y un DataNode mantiene los datos sin procesar. Del mismo modo, en HBase, un maestro HBase contiene los metadatos y los RegionServers los datos. Estos son los servidores que contienen los datos de HBase, ya que es posible que sepamos que en el clúster Hadoop, NameNode administra los metadatos y DataNode contiene los datos reales. Del mismo modo, en el clúster HBase, RegionServers almacena los datos reales sin procesar. Como puede suponer, un RegionServer se ejecuta o se aloja sobre un DataNode, que utiliza los DataNodes subyacentes en el sistema de archivos subyacente, es decir, HDFS.
RegionServer realiza las siguientes tareas:
• sirve las tablas asignadas a él
• Manejo de solicitudes de lectura / escritura del cliente
• Vaciar caché a HDFS
• Mantener HLogs
• Realizar compacciones.
Los siguientes son los componentes de RegionServers:
De la misma manera que en clúster de Hadoop, un NameNode administra los metadatos y un DataNode mantiene los datos sin procesar. Del mismo modo, en HBase, un maestro HBase contiene los metadatos y los RegionServers los datos. Estos son los servidores que contienen los datos de HBase, ya que es posible que sepamos que en el clúster Hadoop, NameNode administra los metadatos y DataNode contiene los datos reales. Del mismo modo, en el clúster HBase, RegionServers almacena los datos reales sin procesar. Como puede suponer, un RegionServer se ejecuta o se aloja sobre un DataNode, que utiliza los DataNodes subyacentes en el sistema de archivos subyacente, es decir, HDFS.
RegionServer realiza las siguientes tareas:
• sirve las tablas asignadas a él
• Manejo de solicitudes de lectura / escritura del cliente
• Vaciar caché a HDFS
• Mantener HLogs
• Realizar compacciones.
Los siguientes son los componentes de RegionServers:
- Registros de escritura anticipada o Write-Ahead logs (WAL): Cuando los datos se leen / modifican a HBase, no se escriben directamente en el disco, sino que se guardan en la memoria durante un tiempo (umbral, que podemos configurar según el tamaño y el tiempo). Mantener estos datos en la memoria puede ocasionar una pérdida de datos si la máquina se apaga repentinamente. Entonces, para resolver esto, los datos se escriben primero en un archivo intermedio, que se denomina archivo de registro de escritura anticipada y luego en la memoria. Entonces, en el caso de una falla del sistema, los datos se pueden reconstruir usando este archivo de registro.
- HFile: estos son los archivos reales donde los datos sin procesar se almacenan físicamente en el disco. Este es el archivo de la tienda real.
- Store: Aquí se almacena el HFile. Corresponde a una familia de columnas para una tabla de HBase.
- MemStore: este componente está en el almacén de datos de memoria; esto reside en la memoria principal y registra la operación de datos actual. Por lo tanto, cuando los datos se almacenan en WAL, RegionServers almacena el valor clave en el almacén de memoria.
- Región: Estas son las divisiones de la tabla HBase; la tabla se divide en regiones según la clave y están alojados por RegionServers. Puede haber diferentes regiones en un RegionServer
Bajar libros gratis desde Bookboon
Quiero compartir esta pagina de libros gratis. Si bien no tiene tantos libros técnicos, esta buena, tiene muchos libros soft, de metodología, Ingles, y tambien hay técnicos.
Dejo link: https://bookboon.com/
domingo, 9 de diciembre de 2018
¿Que novedades nos trae Linux Mint 19.1 Tessa?
Muchos sabrán que soy bastante fanático de Mint, pero no hago un post por cada versión pero esta versión me resulto por demás interesante por pequeños cambios en la interfaz que son un gran paso a la usabilidad:
El gestor de archivos Nemo es ahora tres veces más rápido
Se incluye un nuevo diseño del escritorio mucho más simple, claro y moderno respecto a su predecesor. En la parte de abajo encontramos una barra de iconos más grande incluyendo el botón de inicio (Menú).
Iconos de las aplicaciones ahora se compactarán
Mint 19.1 se viene con todo, si bien no tenemos la versión estable todavía podemos bajar la versión beta.
viernes, 7 de diciembre de 2018
¿Como esta compuesto Apache HBase? Parte 2
Ahora hablaremos del HMaster. Pero antes les tengo que recordar que un cluster Hadoop y Hbase, esta concebido sobre el paradigma maestro y esclavo. Es decir hay un maestro que es el encargado de coordinar las tareas de los nodos esclavos.
HMaster es el componente del cluster HBase que se puede considerar como el nodo maestro a la vez, actúa como un maestro para los Servidores de Región que se ejecutan en diferentes máquinas. Es responsable de monitorear todos los RegionServers en un cluster HBase y también proporciona una interfaz para todos los metadatos de HBase para las operaciones cliente.
También maneja el failover de RegionServer y las divisiones de región. Puede haber más de una instancia de HMaster en un clúster HBase esto lo configuraremos así para tener alta disponibilidad. Si tenemos más de un maestro, solo un maestro está activo a la vez; en el momento de la puesta en marcha, todos los maestros compiten para convertirse en el maestro activo en el cluster. Un nodo maestro ganara y todas las demás instancias maestras permanecen pasivas hasta que el maestro activo se bloquea, se apage o ZooKeeper lo decida. En resumen, HMaster es un componente de coordinación en un cluster HBase, que también administra y nos permite realizar una tarea administrativa en el cluster.
Discutamos ahora que pasa cuando inicia el servidor HMaster:
HMaster es el componente del cluster HBase que se puede considerar como el nodo maestro a la vez, actúa como un maestro para los Servidores de Región que se ejecutan en diferentes máquinas. Es responsable de monitorear todos los RegionServers en un cluster HBase y también proporciona una interfaz para todos los metadatos de HBase para las operaciones cliente.
También maneja el failover de RegionServer y las divisiones de región. Puede haber más de una instancia de HMaster en un clúster HBase esto lo configuraremos así para tener alta disponibilidad. Si tenemos más de un maestro, solo un maestro está activo a la vez; en el momento de la puesta en marcha, todos los maestros compiten para convertirse en el maestro activo en el cluster. Un nodo maestro ganara y todas las demás instancias maestras permanecen pasivas hasta que el maestro activo se bloquea, se apage o ZooKeeper lo decida. En resumen, HMaster es un componente de coordinación en un cluster HBase, que también administra y nos permite realizar una tarea administrativa en el cluster.
Discutamos ahora que pasa cuando inicia el servidor HMaster:
- Se bloquea (no atiende solicitudes) hasta que se active HMaster.
- Finaliza la inicialización.
- Atiende peticiones.
- Limpia los registros al finalizar.
En HBase, hay una tabla llamada .META. (nombre de la tabla en el sistema de archivos), que conserva toda la información sobre las regiones a las que HMaster hace referencia para obtener información sobre los datos. De forma predeterminada, HMaster se ejecuta en el número de puerto 60000 y su interfaz de usuario web HTTP está disponible en el puerto 60010, que siempre se puede cambiar por medio de configuración.
Resumiendo, HMaster es encargado de :
- Supervisa los servidores regionales
- Maneja el failover de los servidores de región.
- Maneja los cambios de metadatos.
- Asignación / desasignación de regiones
- Interfaces todos los cambios de metadatos
- Realiza balanceo de recarga en tiempo de inactividad
- Publica su ubicación al cliente utilizando ZooKeeper.
- La interfaz de usuario web de HMaster proporciona toda la información sobre el clúster HBase (tabla, regiones, RegionServers y así sucesivamente)
Suscribirse a:
Entradas (Atom)